Andrew Means calls for transparency in algorithmic decision-making to ensure proper, equitable practice in service of the public good.
Eric Loomis stood before a judge as he was handed down a six year sentence for “eluding the police.” During sentencing, the judge referenced an algorithm that showed Mr. Loomis was at a high risk for committing another crime and said it was a factor in his decision to give Mr. Loomis the six year sentence.
The judge was using a system called Compas, which was developed by a private company, Northpointe Inc. Compas was purchased by the State of Wisconsin to predict the risk that a particular individual might commit another crime—the higher the score, the higher the risk, and the more likely a judge might feel that a longer sentence is necessary. Mr. Loomis challenged the court’s use of the Compas system; the Wisconsin Supreme Court recently heard arguments in the case and could rule in the coming days or weeks.
At the heart of Mr. Loomis’s case is the fact that the Compas algorithm is proprietary and private, and therefore its exact features and process for calculating risk are not publicly known. As more and more public services rely on algorithms, cases like Mr. Loomis’s raise an important question about how open those algorithms should be and whether they should be used at all.
It’s no question that more public services are being supported, provided, or otherwise shaped by algorithm. Algorithms are being used to terminate Medicaid benefits, render driver’s licenses useless, predict who will commit a crime or drop out of school, identify fraud by hedge fund managers, and catch restaurants with health code violations.
As the public sector is continuously pushed to do more with less, turning to algorithms to improve and support services makes a lot of sense. I’m an avid believer that algorithms really can make life better. They can help provide better services. They can increase the outcomes of social interventions.
The problem arises when these algorithms are masked in secrecy and unable to be discussed, dissected, and validated in the public forum. When the data services and products purchased by cities, philanthropies, and nonprofits are proprietary, the features and models they are using are unknown to the public, and thus not publicly vetted. This raises legitimate concerns.
For example, should race be a factor in determining the likelihood a person will commit a crime? What about gender? Should social media, which not everyone uses, be the data source to identify cases of food poisoning? In the world of data science, the particular data sets and parameters used in a given model can create significant biases that can go undetected if the work is done improperly.
When models are making important decisions that greatly affect human lives—and not just what advertisements we see when we log in to Facebook—we need to make sure that they are equitable, accurate, and appropriately developed and interpreted. This requires transparency and openness.
The movement towards transparent decision support models could actually represent a big step forward for society. While it is obvious that the judge in Mr. Loomis’s case was using a proprietary algorithm, it’s easy to forget that before the State of Wisconsin ever purchased Compas, their judges were already using proprietary algorithms—their own decision-making processes—to deduce the risk of someone committing a future crime. The difference is that those algorithms were purely inside their head, where no one else could inspect them, discuss them, or look at them objectively.
Judges certainly use factors like race and gender as indicators of an individual’s likelihood to commit future crimes. Teachers use socioeconomic status when predicting how well a student is going to do in their class. We all use algorithms to make decisions, but they are masked in our prejudices, biases, and past experiences.
Data-based decision support tools could represent a huge step forward because we can start examining these everyday algorithms. They could be held to higher standards, and we, as a society, could come to some agreement about what should be included and what shouldn’t be.
But the proprietary nature of some data science models isn’t the only thing that makes them impenetrable. Sometimes it’s just the fact that the people buying and using them can’t understand what they do and how they work. Machine learning, artificial intelligence, neural networks—these are complicated topics. The most sought after data scientists have PhDs at the intersection of math and computer science from elite universities (and they happen to be, more often than not, white guys—another imbalance that can contribute to bias). Not only is data science hard to do, it is often hard just to understand. The predictive algorithms being used in social sector organizations can feel like black boxes that we are simply meant to trust.
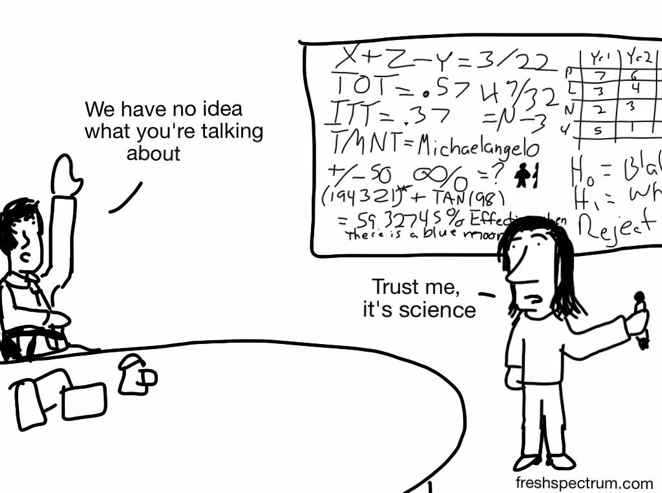
Chris Lysy via FreshSpectrum.com
We need a more data literate society and, certainly, greater data literacy among the leadership of social sector institutions. We need people who can interpret and understand the models and tools being used. We need to be able to engage in both the ethical and the technical.
Algorithms are here to stay. They can legitimately improve the quality of service delivery and better the lives of even the most vulnerable in society. But we need openness and transparency to ensure that they are as socially beneficial and equitable as possible.
My hometown of Chicago has been doing some laudable work along these lines. The city’s Department of Innovation and Technology has developed an algorithm that helps identify restaurants at risk of food safety violations; this algorithm is based entirely on data that the city has made available through its open data portal. In addition to putting the data online, the city also put the algorithm online for anyone to use, dissect, and evaluate. And city officials have also said that if anyone is able to improve the algorithm, the city will adopt the improved model.
This kind of effort, based on transparency and openness, could prove to be an excellent model for the future of algorithms for good. These efforts certainly still face challenges with data privacy, literacy, applicability, and use, but at least such challenges can be addressed openly, and with many voices.
The danger of proprietary algorithms for public good is that we lose the value of diversity. Diversity of opinion. Diversity of thought. Diversity of experience. We must push for increased transparency and openness in the algorithms we are using so that they truly represent and serve the public good.