Experts in technology and data ethics explore the growing use of algorithms and how civil society can work toward better policy for predictive technologies
In October 2018, Digital Impact’s Lucy Bernholz was joined by author of Automating Inequality, Virginia Eubanks, Rashida Richardson of AI Now Institute, and Di Luong of Media Mobilizing Project to discuss the growing use of algorithms in our communities and how civil society organizations can work toward better policy for predictive technologies.
Pretrial risk assessment algorithms are often correlated with race and perpetuate decades of racial, ethnic, and economic bias. With funding from a Digital Impact grant, Media Mobilizing Project is surveying pretrial risk assessment algorithms to expose the bias and transparency issues inherent in many of these tools.
To help ensure that these tools benefit those they are meant to serve, the AI Now Institute at New York University is working with civil society organizations and research institutions to challenge government use of algorithmic decision systems. In her latest book, Automating Inequality, Virginia Eubanks gives examples of how data mining, policy algorithms, and predictive risk models affect poor and working-class people in America.
Civil society groups have a critical role in conducting and communicating research on these technologies. This discussion offers a glimpse of a roadmap for building coalitions around digital policymaking.
Audio Podcast and Transcript
Watch the video or listen below and visit our podcast on iTunes. Follow @dgtlimpact on Twitter for invites to future roundtables. This transcript has been edited for clarity.
[powerpress]
00:00:00 LUCY BERNHOLZ: Welcome and thanks for joining today’s Digital Impact virtual roundtable on algorithmic bias, better policy and practice for civil society. I’m Lucy Bernholz, director of the Digital Civil Society Lab at Stanford PACS.
The Digital Impact virtual roundtable series highlights issues related to digital data and civil society. These conversations are part of a larger constellation of activities that Digital Impact and the Digital Civil Society Lab are undertaking to bring together people thinking about and making happen digital civil society around the globe. We invite you to learn more about the initiatives and opportunities available through Digital Impact, the Digital Civil Society Lab and Stanford PACS. Our primary goal here at Digital Impact is to advance the safe, ethical and effective use of digital resources in civil society.
Today we’re talking about the growing use of algorithms in our communities that has civil society organizations can work toward better policy for predictive technologies. Pretrial risk assessment algorithms are often correlated with race and perpetuate decades or centuries of racial, ethnic and economic bias.
With funding from the 2016 Digital Impact grant the media mobilizing project is leading a project to understand pretrial risk assessment algorithms. The project aims to illuminate the bias and transparency issues inherent in many of these tools. To help ensure that these tools benefit those they are meant to serve the AI Now Institute at NYU is working with civil society organizations and research institutions to challenge government use of algorithmically informed decision-making systems. And in her latest book, which I’ve got to show you and you must go get, Automating Inequality, Virginia Eubanks gives examples of how data mining, policy algorithms and predictive risk models affect poor and working-class people in America.
Over the next hour our panel will discuss the social and economic implications of these predictive systems and the critical role of civil society and civil society organizations in conducting and communicating research on these technologies.
Let me start with a few housekeeping details before we start. For everyone but the panelists your microphone will be muted for the length of the discussion. We do want to hear from you so please use the comment function on your control panel which will submit your questions to me and I’ll pass them on to the panelists. The discussion is going to be recorded and it will be shared on the Digital Impact podcast channel on iTunes and at digitalimpact.org. You can join the discussion on social with #DataDiscrimination and subscribe to our mailing list.
I’m very pleased to introduce the panel, we are joined by Virginia Eubanks who’s an associate professor of political science at the University of Albany, the State University of New York at Albany. Sorry as a New Yorker I should get that right. And the author, as I said, of Automating Inequality. Rashida Richardson, go ahead and wave hi Rashida, thank you, is the director of the policy research for the AI Now Institute at New York University. And Di Luong is the research and policy organizer at the Media Mobilizing Project. Thanks to each of you for joining us today, now let’s get started.
[3:58] Virginia, I’m going to start with you, your book Automating Inequality paints a powerful picture of how digital data are used in the United States and have been for a long time, probably longer than most people realize in ways large and small, visible and invisible. We’ve handed over at least part of our decision-making authority from people to machines. Automated eligibility systems, ranking algorithms, predictive risk models are all examples of how these technologies are standing in for human decision-making. The tools are trained on data that all of us generate through social media, every time we interact with our government to apply for something or if we pay a parking ticket or something. And increasingly through sensors and the Internet of Things. You’ve described it as a data analytics regime that we all live under, but we don’t all experience it the same way. Can you explain what you mean by that — what the regime is and how we experience it differently? And then you’ve got a very powerful phrase called the “digital poorhouse,” which is probably worth introducing to people as well.
00:05:11 VIRGINIA EUBANKS: Sure. Thanks so much for having me, I’m really excited to be here and hello to everyone who’s participating. I’m just so excited to be on this panel with Di and Rashida whose work I admire and respect immensely, so thank you so much for having me here to be part of the conversation. I use this phrase the digital poorhouse in the book and that phrase is meant to contextualize these new tools and their deeper policy history.
Often when we talk about new technologies, we treat them like they’re the monolith from 2001: A Space Odyssey, like they just come from nowhere, they land on blank ground and change human history forever. But of course, that’s not how technology works. It comes out of human culture, it’s built by people, it’s shaped by the society it emerges from and goes on to shape that society.
The reason I use this phrase the “digital poorhouse” is to talk about what I think of as the deep social programming that goes into systems that are specifically aimed at social services. So, in the book I talk about welfare, Medicaid, food stamps, homeless services, and child welfare or child protective services. Because I think that we also have a tendency to talk about these tools as if they affect us all in the same way. That because they affect us all in the same way, we can all live under the same set of solutions, like increased privacy or informed consent. And that becomes a real issue when you look at these systems where these tools are being used in what I think of as “low rights” environments — places where people don’t necessarily get to fully consent to the kind of interaction they have with these tools.
Data justice project Our Data Bodies works with local communities to investigate how digital information is collected, stored, and shared by government and corporations.
Everyone is not a consumer of these tools, sometimes people are in situations where they can’t meaningfully say no. So, for example, you can [decide not to] collect food stamps that you’re eligible for and by not collecting food stamps not give the government just reams and reams and reams of information about your family. But if you don’t have enough food in the house it actually means that you’re vulnerable to a child protective services investigation that might result in your child being removed and put into foster care. So that’s not really meaningful consent of interacting with the data collection that goes on around food stamps, for example.
So, I think sort of placing these tools in context that in social services these digital tools go back at least until the 70s and early 80s, and have been collecting information on poor and working-class families for a really long time. And so that’s part of the intention of using that phrase the digital poorhouse is to go back into our history to put these new tools into policy context.
00:08:13 LUCY BERNHOLZ: It’s also really important and I want to flag this for questions and for the rest of the panelists is that we’re talking about 40 years of data collection — 40, 50 at least years of data collection here that’s part of this overall social system as you describe it. So, this is really not a new phenomenon. Let me turn now Di to get your thoughts on some things.
A couple of months ago Joi Ito who’s the director of the MIT Media Lab wrote quote, that we’re using algorithms as crystal balls to make predictions on behalf of society when we should be using them as a mirror to examine ourselves and our social systems more critically. Your team at Mobilizing Media Projects seems to be trying to do something like that — putting a mirror up to the criminal justice system in Philadelphia in particular about the use of these tools. Can you tell us a little bit about what both the City’s trying to do with its pretrial risk assessment algorithm and how the work of your organization and your research is informing its design and implementation?
00:09:22 DI LUONG: Yeah, thanks so much for inviting us to this virtual roundtable. Holding a mirror to our social system is an apt and poetic description of algorithms because at the Media Mobilizing Project we recognize the potential flaws and how data is collected, and how it can skew results and perpetuate biases, especially during a pretrial period when an individual has not even faced trial or even been convicted. And so, when we found out about three years ago that a pretrial risk assessment tool was going to be developed in our city, it was impetus for us study nationally what’s going on. So, we created a survey and we also looked at a lot of the governmental documents and academic reports and industry information that is just out there.
“Algorithms trained on racist data have big error rates for communities of color,” writes Media Mobilizing Project’s Hannah Sassaman. “That person and their allies should have the right to face what that algorithm says and tell their unique, human story.”
We accumulated a data set of over 150 pretrial assessment tools that are being used around the US. And our goal is really to create a comprehensive clearinghouse for the public to review the component of each tool that’s used in their city and then compare it to how other tools are used in a neighboring city or a neighboring state. And we really hope that this research could identify, better position the number of counties that are using a particular product, or whether a state is using a statewide tool as opposed to a state that’s using a myriad of tools.
The New Mobilizing Project designed our research framework with like a surface-level audit cataloging the designers of the tool, the name of the tool, and also how to even define recidivism. We then wanted to emphasize community impact by asking through interviews and secondary analysis, does it increase or decrease a jail population once a tool has been implemented? And [we] also looked at the policies and practices of how these tools are implemented.
00:11:18 LUCY BERNHOLZ: Okay thank you for that and I’m going to introduce Rashida and ask her something, but I want to come right back to that research as you described it. But Rashida, first of all the AI Now Institute has joined with a number of other organizations to challenge the governmental use of algorithmic decision-making systems across a range of institutions, including criminal justice. So, it’s not just about pretrial risk assessment and as Virginia has already said, it touches a number of different elements of life. One problem that exists is the lack of oversight which tends to result in weaker protections for vulnerable populations. But tell us a little bit about the coalition that your institute is working with and how you’re trying to influence that issue.
00:12:04 RASHIDA RICHARDSON: So, again thank you for having me here. The work that we’re doing is kind of multifaceted. I’ll start with a few examples. We’re working with a group of local and national organizations in New York City to influence the process where there’s a task force that was created by a law earlier this year to look at government use of algorithms in New York City. That task force was appointed in May and they’re supposed to release a report with recommendations at the end of 2019.
AI Now Institute’s Algorithmic Accountability Policy Toolkit provides legal and policy advocates with a basic understanding of government use of algorithms.
One thing that I learned through the legislative process of getting that legislation passed and also through this process of even getting the task force announced is that both people and government and the advocacy community knows that these issues are important, but no one really understands what to do about it. And that’s not necessarily like a “what to do” but we are working with a number of groups who understand economic justice issues, understand racial justice issues, criminal justice, housing, healthcare, you can name a full list. But they may not have access to enough information to understand what the best policy solution is within government. And then the reverse a lot of bureaucrats within government may think they have the best solutions to their systems, but they don’t necessarily involve people who are impacted to understand that maybe even the goal of a system is not the best approach.
So, our focus with this coalition work in New York City is to try to just move this process along, understanding that, or trying to work under the assumption that everyone’s acting in good faith, but may not know the best approach. So how to make sure that people are sort of sharing ideas and one way we did that is in August we released a letter to the task force that is about like eight pages of recommendations that are tied to the provisions of the law. And it’s in part to help the task force in that like these are some of the things that ultimately they need to make recommendations about. But it was also to help spur conversation within the community of like here’s some ideas and these don’t have to be where we land a year from now, but at least it’s a starting point for thinking through what should a standard be to redress harm that’s been identified in the system.
And then I’ll give one another in that earlier this year we held a convening called Litigating Algorithms and we just put a report out of its findings. And that was an effort to work with national and local organizations that have litigation capacity to talk about strategies that work and don’t work. Because I don’t think this is an issue that can just be legislated, I think even if you do legislate there are going to have to be some challenges to where that legislation falls short.
So, our goal is to build research that can help inform these processes, but also work on multiple levels of how policy decisions are made to make sure that the lawyers who know what they’re doing in the area are informed enough, but also they’re aware of sort of where the discourse is going to make the best recommendations.
00:15:12 LUCY BERNHOLZ: Great. Well we have jumped right into it, this is perfect because all three of your introductory comments — and I’m going to ask Virginia to respond first to what I’m about to say — have made it very clear that not only are we not just talking about technology we’re talking about technology embedded in social systems. And there’s a kind of a recursive relationship there. But we’re also talking then about how do you make policy, how do you think about this, and how do you engage the right people, the people who are affected in these issues in that work. But I have a question sparked, so a direct question to Di — and then I go to Virginia and ask her to jump in, and Rashida you can follow in on that — Di, you talk about what at first glance sounds like oh we’re going to collect information about all jurisdictions that are using these tools. And as a researcher myself the first thing that comes to my mind is oh yeah, do they tell you what they’re using. So, in this weird way that transparency doesn’t always work to the benefit of the people, how are you actually finding what governments are, you know, whether that’s the city of Philadelphia or you know the state of Pennsylvania or the US [inaudible], who’s reporting out what’s being used and how much information is available to the public about that?
00:16:29 DI LUONG: Yes, we aim to collect as much information as possible that we can find online, and also take stock in what we have. And when there are gaps and that is when we develop a survey and then push for further inquiry into getting the feedback from pretrial service agencies or at least the implementers of these tools if that makes sense. And then also for information that an implementer does not know or the information that’s not available online, then that is when the work of civil society, the work of grassroots advocates come in and really demand to see the source code and really demand to see. We welcome an AI institute for help and also academics, like Virginia and you as well to help us really push and pressure for this information.
00:17:25 LUCY BERNHOLZ: Well and I want to just pick, I mean so, clearly there’s one role of civil society here is trying — as usual, as often as the role for civil society — to hold governments to account just about what it is they’re using, right? In a democracy, we have the right to scrutinize power and one of the core issues here is whether humans can know what kind of decision tools are being made — are being used — to make decisions about them. But Virginia, it’s much more complicated than that. It’s much more than oversight, it’s much more than transparency. Help us think through the other elements of how to really kind of democratically assess the use of these tools for these kinds of decisions.
A People’s Guide to AI is a resource for understanding the impact of artificial intelligence on society.
00:18:10 VIRGINIA EUBANKS: Yeah, so I think we and you know for good reason we’ve really focused a lot of attention on things like accountability, transparency, and sometimes on the edge of human-centered or participatory design of these systems. I think all of those things are absolutely crucial to systems that are making political decisions for us in a democracy, let’s just say that’s absolutely necessary. But I also feel like that’s kind of step zero and I mean it’s like the lowest possible bar for decision-making in a in a democracy — that we like know how the decisions are being made, that they’re accountable to some kind of public process. And that we’re involved in some way in them. Like that’s a pretty low bar to get over and I think we can do much better than around these new tools.
So, I’m really excited to hear Rashida talking about redress because that’s one of the things that has come up a lot talking to people in communities who are directly affected by these technologies, it’s not just like how do we understand them or have some oversight around them, but also like if it harms us what we do. And hi to your cat.
So, redress is really important. But one of the things that I want to just make sure that I list is one of the things that really came up when I was doing my reporting in the book was around the way that these tools often dismantle established rights that are really important to people. So just for example, Lindsay Kidwell in Windfall, Indiana, in the chapter that’s about the Indiana automation in my book, lost her Medicaid during this automation of the eligibility system for welfare, Medicaid and food stamps in Indiana in 2008. She was very sharp and had a really good advocate and so she reached out and asked for what’s known as a fair hearing. And a fair hearing is this incredibly important right was won specifically by welfare rights organizers in the late 1960s and early 1970s that says there has to be an administrative law procedure that’s outside of the welfare office to decide whether or not decision-making is fair and correct.
So, she asked for a fair hearing. Several weeks later she got a call from a guy on the phone who said he was calling to schedule her fair hearing for her Medicaid. And then he said, you know, “I’m looking in the computer, I’m seeing what the judge will see. We see there’s no evidence that you’ve cooperated with establishing your eligibility for this program, so please just cancel your fair hearing right now.” And there’s a way that these tools often, because they have this veneer of objectivity and neutrality, get used as like the final truth in any case. And then there’s pressure for people to not go through the due process that they’re allowed and really should follow up on.
And so, I think the rollback of rights, the rollback of previously established hard-fought rights to things like due process are really, really important to keep on the table. And I think the reason that we don’t always keep these really direct important issues at the top of the agenda is that we’re not always talking to folks who are most directly impacted by these systems. I really think that has to be absolutely step zero in this work is to look at the places where these tools are most directly impacting people’s survival, health and family integrity, and asking them what they want and what their most important concerns are.
00:21:56 RASHIDA RICHARDSON: Can I [Inaudible] add some color to this, in that I think it’s really important everything Virginia just said and I’ll get to like how that can be incorporated in a meaningful way. But it is important to have the voices of those who are actually affected by these systems at the table speaking to whether even the current use of an algorithmic system is working or how it should be revised when we do have these horrible cases of finding out they don’t work. Because I’m finding in some of the research that I’m doing that a lot of governments are kind of using this alternative approach of using civil society groups as a means of saying that they’ve included vulnerable community voices. But then also not even allowing those civil society groups to have meaningful access to the process.
Tech developers’ own biases and prejudices can result in reproducing inequities. But can tech also work to mitigate social division?
So, a lot of the times they’re being brought in after a system has been acquired, almost right before it’s about to implemented or while it’s already being implemented just so they can say hey check we asked some groups to give feedback. And then that is used as justification for a system and that I think just needs to be called as the problematic process.
But then also I wanted to mention that earlier this year we put out report called Algorithmic Impact Assessments, which is a governance framework that we hope can be implemented to create better transparency, accountability, oversight and community engagement throughout the process. For that framework we drew on other existing impact assessment frameworks. So, in the United States we use environmental impact assessments before a large highway is built to assess whether or not one, it’s going to have environmental impacts but two, if it’s going to have social impacts that can’t be redressed in any way. And it’s a way of getting community voices in early, but instead of like wasting a lot of time walking through how the framework works I think it’s just important to mention that part of the process is forcing governments to be public about when they intend to use a system, and that this process would happen before it’s implemented, so it gives an opportunity for all of the experts in the world — and I mean that as people who are affected by the systems — experts of the economics of a system and so on to have input to hopefully either stop something before it happens so you don’t have to have litigation bringing this about. Or find ways to mitigate harms where if a system could actually be value-added in the long run you can find ways to adjust some of the problems early so you don’t have to have negative outcomes at all hopefully.
00:24:37 LUCY BERNHOLZ: So, let me just ask if I can — and we’re getting a lot of additional resources being suggested, both from panelists and from people listening and requests for the things that you’re pointing to. So, I want to assure everybody on the call that everything that’s being mentioned we will in the final writeup of the conversation and the podcast and the transcript and all of that we’ll be collecting all those URLs and resources. So that’s also a prompt to people listening to shared things, there’s lots of other ideas out there that you’re a part of and folks are starting to share those. I do want to ask though — whether or not we go into it in depth or not — do the three of you on the call feel like among at least this group, there is a consensus about the complete policy process that should be followed to ensure the rights of those affected by these decisions, the recourse, the shaping of them? Like do we know how to do this right or are we still trying to figure that out? And Rashida I’ll start with you and then just Di and Virginia if you want to comment on that.
00:25:55 RASHIDA RICHARDSON: One thing we learned from our litigating algorithms event is that due process challenges still work to a certain degree. But I don’t think that’s the best approach in that it requires someone to be harmed in some way — to either have some type of liberty harmed or for there to be some type of procedural government harm — and I don’t think that’s the best mechanism for addressing public policy decisions. I think there needs to be something up front and I also think there needs to be some form of enhanced due process rights, so we’re not just relying on the state’s administrative law to correct a harm or waiting for some like large catastrophe to happen for there to be litigation. One is our algorithmic impact assessment framework obviously, but that’s more of a holistic approach.
Anatomy of an AI System illustrates the Amazon Echo as an anatomical map of human labor, data, and planetary resources.
I also think some of these issues could be corrected with some more upfront transparency from government. It’s sometimes hard to challenge a system if you don’t know it was a system that made a decision that affected you. And that was in fact one of the recommendations in the letter that I mentioned earlier was for the government to archive all of the decision systems that they would be public about so that way you know, part of the process is you need a definition so you’re not just capturing like Excel formulas or other sort of, even though there are some harmful Excel formulas, but you’re not capturing systems to then make the argument for this is too burdensome, too easy for government agencies. So, once you sort of define when we’re looking for or the types of systems we want to capture there should be some type of public list of what was included and what was excluded so that way you have an idea as not only what’s out there and what is being used that may affect you. But also, when decisions are made to exclude a system that is harmful there’s a way to also challenge that, the exclusion of that system. So, I think just more transparency up front could be the first step in helping, but there’s a lot of other sort of bureaucratic problems that would have to be fixed to enhance [inaudible] procedures.
00:28:13 LUCY BERNHOLZ: Great, thanks. Di, did you want to jump in on that?
00:28:15 DI LUONG: Yeah, thanks. I think as a social justice community organization we want to demystify a lot of the technical jargon that’s often used when discussing automated decision-making tools used by the government. And so, we want to turn data points into human stories and that is part of the bulk of our work.
00:28:36 LUCY BERNHOLZ: Great, thank you. Virginia.
00:28:38 VIRGINIA EUBANKS: Yeah. I just want to underscore what is Di is saying and that one of the things that came up in my reporting was really across the board for all the different systems that people were interacting with. One of the things they found most frustrating and upsetting was actually feeling like their whole human stories were being flattened to a set of data points and then made these really important decisions about their lives. Folks felt that was really dehumanizing and locked them into like patterns of their past or patterns in their community in ways that they just found deeply troubling. And so, Di, I just want to underscore that people that I spoke to felt very much like that was part of the problem was this flattening of whole human beings into sets of data points.
But I think one of the things that we haven’t figured out yet and we really need to be thinking about is meaningful ways to say no to a system we think’s going to be harmful before it rolls out. For example, I’m still on the fence about this one, but I’m becoming more and more convinced that because of the nature of the data we have available and the need to use proxies and how complicated the social process of child protection is that we shouldn’t be using predictive tools in either intake screening or in decision-making in child protective services. I just think it’s problematic to the core in some very deep ways. But there doesn’t seem to be a meaningful way to say, “you know what no, we’re just not going to use it. If we say no to this, don’t do it.” I really think that we need to spend a lot of time and energy developing those tools for helping communities say no to tools they just do not agree with and do not want to see developed.
FURTHER READING
H.R.4174 (Foundations for Evidence-Based Policymaking Act) aims to improve data use in the federal government.
H.R.3895 (Smart Cities and Communities Act of 2017) establishes programs for implementing and using smart technologies and systems in communities.
S.1885 (AV START Act, or American Vision for Safer Transportation through Advancement of Revolutionary Technologies Act) aims to support the development of highly automated vehicle safety technologies, and more.
H.R.3388 (Self Drive Act) establishes the federal role in ensuring the safety of highly automated vehicles.
00:30:24 LUCY BERNHOLZ: That’s a really important point. I’m glad you brought it up, it’s something I’ve been wondering about a lot. Rashida or Di, do you have any comments on that or examples where communities or someone has stood up and said no we’re not going there, it’s inappropriate, it causes more harm than it does good or whatever? Are there examples where this has been prevented?
00:30:52 DI LUONG: Virginia talked a little bit about the proxies or at least the items used within an algorithm that are proxies for socioeconomic situations. For example, using the use of housing which is someone who rents a home is considered riskier to someone who owns a home or income or job instability is seen as somehow a predictor of recidivism in the future. And that is something we’re particularly concerned about. And there has been a resistance locally in Philadelphia from community groups and we had a rally in front of City Hall in order to resist against the use of these tools.
00:31:38 LUCY BERNHOLZ: That’s interesting. And someone just submitted a story from a Philadelphia paper about a successful effort to at least change the timing of the use of these tools, so we’ll make sure to share that as well. Rashida, I’m sorry I cut you off.
00:31:55 RASHIDA RICHARDSON: I was just going to mention that there was also an effort in Massachusetts where they were doing a mask criminal justice reform effort and then through the legislative process there was an effort to push forward sort of supplanting the existing process with the use of the risk assessment tool. And there, there was an advocacy effort amongst researchers from MIT and Harvard that pointed out the problems with just using a risk assessment tool instead. So that helped slow down the process. I think what ended up happening there is that the bill was revised, so the bill hasn’t fully passed into legislation. But that effort from the researchers was heard and then did force legislators to rethink the effort they were planning to move forward with.
00:32:50 DI LUONG: This reminded me of recently in Boston of parents who resisted against a tool used to determine bus routes. Because that algorithm is done by MIT and that was, so you see parents holding up signs that say “families before algorithms.”
00:33:10 RASHIDA RICHARDSON: Yeah, actually if I could expand on that because there were two. Boston is an interesting test case because there’s been two failures recently that there’s been writing about. First, I think in July northeastern researchers issued a report about a school desegregation algorithm that was implemented in Boston back in 2014 through 2015, so it was sort of a post hoc review of what happened and in short, it didn’t work. And then the same happened with what Di’s talking about and that was an algorithm that was designed by MIT students to change the school start times and bus routes so that way high schools could start later. Because what they found is that one, in general high schoolers performed better if they had more sleep. And most of the high schools in Boston that had the better start time were more in the whiter, more affluent areas, so they wanted to try to redistribute that. But in that example, I think that was where you didn’t have all voices at the table and what were you optimizing for.
So, when they did ultimately find the algorithm that sort of balanced all of their interests there was huge backlash amongst all of the parents, but it’s interesting to see the two factions. One was the white affluent parents that were angry that their school times got adjusted. And then the other faction was led by the NAACP and the lawyers’ committee and that was for a lot of the lower income parents of color where they were working lower wage jobs where they couldn’t change their schedules. And even though the algorithm did result in their students getting more favorable times or evenly distributed start times, that’s an example of the process where if you had voices at the table earlier on it could’ve been optimized in a way that could have made more people happy, rather than a complete backlash that I think resulted in the superintendent stepping down.
00:35:18 LUCY BERNHOLZ: Interesting. You know, we talk a lot about including people as informants or you know resources to the decision makers. So obviously, if our decision-making bodies were more representative of the people that would also be helpful. Virginia, I cut you off and then I do want to [inaudible].
00:35:36 VIRGINIA EUBANKS: This is really a great place to be, to be talking in, and I think it’s not just about representing voices, but also giving people power, right? So, this idea of — we’re supporting the power that people already have. So, this idea of the ability to say no. One of the places I’ve come into contact with that is from Shekhar Narayan from Seattle, of Washington ACLU and Seattle’s recently passed an ordinance, the surveillance ordinance that basically ensures that the public has an opportunity to know about, talk about, and weigh the costs and benefits of all new surveillance technology before the city obtains it. And this is the very first step and very preliminary but it seems like a promising process for making sure that people have the opportunity to think about these tools that will really affect their day-to-day lives, and the ability to say no to them if they decide that the trade-offs aren’t worth it.
00:36:44 LUCY BERNHOLZ: And a big part of that is you know we’ve used the metaphor of a highway. Highways are pretty visible. We really need to make the invisibility of this infrastructure go away, it can’t be that kind of visible.
I’m just going to switch if I can to the questions from the audience, putting aside the ones we had talked about because there are some great questions here and I want to get as many of them in as possible. So, I’ll ask the question, if a panelist would just sort of point up a finger if you want to jump in because they’re not really specific to anyone in particular.
One of the most incarcerated cities in the country expects predictive technology to improve on its human-run system. But it could make things worse.
So, there’s two related questions about both other domains that this kind of reimagining, have a complete process of making decisions gets applied to AI might be applied to the way these tools are used in the financial industry where it’s less out of the government’s face and more privatized but have enormous implications for the way we live our lives. So, if there’s any comments on that. But there’s also a comment related to that which is I guess I’d put it into the bucket of there are certain kinds of algorithmically informed decision-makings we should be more worried about than others. And the question, the way it’s raised here is that those that are designed for systems that are punitive in nature, are they distinct from, should we think about them differently perhaps from those that might be a part of decisions that are about trying to improve a set of outputs? So, I guess the general question I’d ask you is are there actually different kinds or different tiers of decision-making using algorithmic inputs that we want to triage and avoid or are we concerned about the entire gamut? Anybody want to jump in?
If that’s not a meaningful distinction, I think that’s important to share with folks. And the next question I’m going to ask is going to be very familiar to all of you and it’ll be right in line with this question. But Rashida you raised a finger.
00:39:08 RASHIDA RICHARDSON: Yeah. I guess I was hesitant to answer because I think what I’m noticing in a lot of the conversations I’ve been in is that there’s urgency around certain uses because obviously life and liberty are something that, are the consequences. So, it’s like we should be trying to address those types of systems sooner. But I get really concerned when there is an attempt to prioritize some harms over others because I don’t think we know enough to know the full consequences of a lot of these systems. Some are very grave and we can understand that just from understanding what it’s optimizing for. But I think when you try to triage, then it will make other harms seem as if they’re not as urgent when it could just be we don’t have access to enough information to understand what those consequences may be.
And I know we keep harping on transparency as being a major issue, but that is the concern and especially around government uses, part of the reason why this New York city task for was developed is because most people in the city council and within government didn’t know where these systems were being used and we still don’t know. That’s one of hopes of the task force. So, it’s hard to even triage if you don’t fully understand the problem.
00:40:30 LUCY BERNHOLZ: Yeah. And I want to just build on that I think from one of the questions that’s here as well. It seems to me one of our very typical societal choices with things like this is we like to experiment on young people. And the education system for decades has been rife with variations of this conversation. And we seem to just go through cycles of being surprised at how poorly we’ve made decisions over time. So, I hear from Rashida that triaging and prioritizing harm, especially given the unknown nature of the decision-making environment over time is really probably not in our best interest. Di or Virginia, you both raised your finger right on that. Di?
00:41:20 DI LUONG: Thank you. I think MIT never set out to fight against the machine because we are always asking for scrutiny over human biases that goes into a design and provide inputs into these machines. And so that’s what our research really focuses on, so the inner workings of the algorithms. Because when the source code and data is not available, what do we know and what could help us gain more power.
00:41:46 LUCY BERNHOLZ: Virginia.
00:41:47 VIRGINIA EUBANKS: One of the things that I think is a really important move is moving from talking about intentionality – like do they mean to screw people — to impact — are they screwing people. Because I think the intent question is not that interesting and I think the impact question is really, really important.
And so, one of the problems with saying right like is it just these tools and punitive programs is that not everybody understands programs as punitive. You know for example, the administrators and the designers of the Allegheny Family Screening Tool — the statistical model that’s supposed to predict which children might be victims of abuse or neglect in the future — they clearly don’t see child welfare as a punitive system. And I understand why. The system actually also does provide a lot of supports for families, but families also experience it as deeply punitive and punishing and threatening to their family integrity. So, there’s a very serious question there about point of view of like, whether a system looks punitive or not depends on where you are in the system.
The Seattle Surveillance Ordinance aims to provide greater transparency to the City Council and the public when the City acquires surveillance technology.
And I think one of the things that’s really important there is I think we pay a lot of attention for very good reason to these tools in criminal justice, both in policing, the role in police violence and of police accountability. Also, in the criminal justice system and in the courts. And one of the invitations I was hoping to offer with Automating Inequality was to see those processes of policing as happening outside law enforcement as well. So, we also see those processes in welfare, we also see those processes in homeless services, we also see those processes in child welfare.
So, while it’s absolutely crucial as my colleague Mariella Saba from the Stop LAPD Spying Coalition always says, it’s crucial to keep your eye on the badge but policing wears many uniforms. And so, the systems that I look at are systems that folks outside who don’t have first-person direct experience with them often don’t think of or experience as punitive. But folks inside the system will tell you that they are and that the technology plays a key role in that. So yeah, I think intention is less important than impact.
00:44:09 LUCY BERNHOLZ: Perfect. And I’m just, we’ve got 15 minutes left and many, many questions here. So, I’m going to go through them quickly. I’ll ask if you don’t get a chance to respond among the panelists we usually try to actually ask you to do this in text afterwards, so there will be some follow up. A question I know you’ve all been asked many, many times which is, shouldn’t we look at whether the biased algorithmic decision-making is biased than biased human making of decisions. And I’m just going to put the question out there and ask one of you to respond to it and the other two to respond in text. I’m sure you get asked this all the time.
00:44:47 VIRGINIA EUBANKS: I have a very fast response to this, which is I have a very smart friend named Joe Soss, who’s a political scientist, who says discretion is like energy, it’s never created or destroyed, it’s just moved. So, when we actually say we’re removing discretion from these processes it’s important to reframe that and say, where are we moving the discretion to? So, for example, in the child welfare case we’re moving discretion from frontline call screeners who are the most racially diverse, the most female, the most working-class part of that workforce, to engineers and economists who built the model who are not as diverse as female or as close to the problems that they’re supposed to be solving. So, I think it’s really not about removing bias, but moving it and it’s important to track where the control over bias is going in these systems.
More than a hundred organizations signed “The Use of Pretrial ‘Risk Assessment’ Instruments,” a shared statement of civil rights concerns about the use of predictive tech in the criminal justice system.
00:45:43 LUCY BERNHOLZ: Fantastic. And I will like I said ask, I give plenty of opportunity for Rashida and Di to chime in on that. So, the next one I’m going to ask either Rashida or Di to answer, which is it speaks a little bit to what was said earlier about data proxies. And the question is, how important is it to institutionalize mechanisms to capture the provenance relationships between data training and inputs, algorithms and decisions? Where does that fit into the mix?
00:46:17 DI LUONG: A judge for example might see the end result after or the final score that is spit out of an algorithm. And the judge thinks oh all the variables are working together like Destiny’s Child when in reality maybe one of them is Beyoncé. And that is really sort of weighed more heavily than the other components.
00:46:41 LUCY BERNHOLZ: Right. Again, Virginia Rashida you’ll have a chance to jump in. Rashida, I’ll ask this question specifically of you. The person asks, there are a number of bills currently under review that deal with the use of AI, IOT and the use of private data. And then they list a number of them that from the numbers that are given I think these are all legislation-pending at the federal level. Are these bills enough to address algorithmic inequality concerns, are there any efforts made by the panelists to collaborate with the federal government to strengthen legislation to protect civil society and consumers of public services? And then it lists a couple of House bills, a couple of Senate bills.
00:47:28 RASHIDA RICHARDSON: So, I can’t say I’m familiar with all these because some of these are broader smart city bills. But I did recently do just a search to see what legislation is being proposed in the federal government around AI and algorithmic systems. And a lot of the federal legislation — sort of carving out maybe some of the smart city efforts that I’m not as familiar with — are focused on more of the keeping the US competitiveness globally at speed. So, it’s more focused on investments in AI and researchers or trying to understand, there’s a few bills that are trying to look at labor impacts. But none of them are really focused on seeing the implementation as a problem. They’re more on how can we continue to develop at the speed that we are developing, to stay competitive and to make sure our economy is continuing to grow and benefit from its use. Not so much on the are we even using or like are current implementations harmful in any way.
We are trying to do more education in DC with legislators so they understand the sort of harm part and the impact part. So, the conversation doesn’t become completely usurped in this we need to be a global competitive force and maintain the advantages that we have. But it’s hard to re-steer that conversation because so much, if you look at Canada, France, EU, a lot of the legislation or conversation is very focused on how can we remain competitive, how do we maintain the talent that we do have. I think there just needs to be refocus and it’s hard to do that when so much of the conversation is focused on development.
00:49:23 LUCY BERNHOLZ: It’s fascinating because it reminds me then of the way the privacy conversation has for decades been framed as privacy versus security. What I hear you saying is this is a conversation that’s being framed as economic influence globally, leaving aside justice.
00:49:46 RASHIDA RICHARDSON: Yeah. Pretty much it’s like defense — like labor is maybe the only area that I’m seeing some type of reflection on impacts beyond the economy, and even that is an economic argument. So, it seems like justice may be in the title of something but I don’t think that’s the focus of the bills.
00:50:09 LUCY BERNHOLZ: Which raises something we haven’t touched on here and maybe one or two or three of you want to comment on, which is where are these algorithms coming from? I mean are they being developed within the public sector, are they products being sold to governments? Both, is there a balance?
00:50:23 RASHIDA RICHARDSON: It’s both. I’ll stick with local and state governments because the federal government is way more complicated. But it’s both in that you have some agencies and especially in New York City because it has the largest municipal budget in the world. Some agencies are just bringing in vendors like IBM Talent Share to help develop in-house systems. So, if you have a big agency like the NYPD they can just say this is what we want developed and bring in someone to help them develop that.
AI Now Institute’s 2017 Symposium Report provides an update on the landscape of AI issues.
A lot of other governments that may not have as robust of a budget sort of buy off-the-shelf systems so that’s from vendors that are developing these system and sort of marketing. But I want to be clear that it’s not always government money that’s being spent and this is in part why there’s a transparency issue is because government procurement is its own problem. So, you have systems being funded in different ways. Some systems are being bought with government funds, some are being purchased by third-party organizations. So, you either have philanthropic organizations giving money to an agency to purchase something. And in New York City that’s like the NYPD Foundation, which bought the most recent body camera system, there’s where third-party money is being used. You have federal grants, so you could have the DOJ or other federal agencies that are giving money to state or local governments to purchase these systems sometimes for pilot projects, sometimes to just use generally.
And then in some areas you have asset forfeiture money being used to acquire systems. And then some vendors give these systems in kind. Say there was a lot of reporting on Palantir giving the system to the New Orleans Police Department where even their city council was not aware. And that’s not exclusive to Palantir, you also have the Arnold Foundation, which has a risk assessment tool. They’re a foundation so they’re giving out their system for free.
And then you have some vendors that are smaller, not big-name vendors, that are trying to get into the market. So, they may offer a system for free to one government so then they can go — I’ll keep using New York as an example — you can have a vendor that goes to the NYPD and offers a system for free. So, then they can go to the Houston Police Department and say hey we’re already partnering with New York City, now can you pay for this or we’ll offer it at a reduced fee.
And I think that’s part of why we such pervasive use of these systems is especially if you’re dealing with a cash-strapped district it’s hard to say no to a promise of efficiency at no cost or low cost. I just want to give that full picture because it’s kind of a myriad of issues that feed into why we’re seeing these systems used in so many areas.
00:53:12 LUCY BERNHOLZ: And a really important area maybe the research has been done, maybe the journalism has been done to reveal the economy of this, which important to point out that civil society by nature is diverse, fragmented and contentious. And as Rashida just pointed out, is not only on one side of this issue.
Mortgage lending in Philadelphia has brought attention to technology’s role in weakening the city’s race relations.
Two last questions, I’m going to tee up the second one for you because we’ll use it to close it out which is a question about the work that each of you do if you have ideas essentially about how to make the conversation, the research, the advocacy, the policymaking more cross-disciplinary and less of a technological effort. It predicates the question in some of what’s been learned in the EU, but I’ll just put that as the final question is going to be what can we do collectively to make it a more inclusive and a much broader set of expertise involved in the conversation.
But before I get there, a question you may have been asked before, is there a trade-off between accuracy and explainability, and how should members of civil society, members of the public think about that? So, is it true and how should we think about it?
00:54:33 DI LUONG: In the pretrial context, transparency supplements accuracy. Auditing and evaluating an algorithm by an independent third party is essential to ensuring that algorithm is not only fair but it also holds up our constitutional values and laws.
00:54:52 LUCY BERNHOLZ: Great. So, it’s not a trade-off they’re in line. And again, it’s the way the conversation gets framed that needs interrogating. Virginia, you look like you wanted to add something?
00:55:00 VIRGINIA EUBANKS: Yeah, I was just going to say that’s how science is supposed to work right, like science is supposed to be accurate because it is transparent and open and you replicate experiments. And so yeah, to do anything else is junk science and not the policy either.
00:55:15 LUCY BERNHOLZ: Great. Rashida, anything to add?
00:55:17 RASHIDA RICHARDSON: No, I agree.
00:55:20 LUCY BERNHOLZ: Fantastic, great, great. We got one more question coming in here let me see if I can get to it. So, there’s a question about the role of civil society. A difficult question always because it’s not one thing. But the question actually says, you know, given the dynamic nature between bias in the real world and bias in the cyberworld and internet world and algorithmic world, shouldn’t civil society be focused on reducing discrimination, bias, prejudice, racism in the overall, not just focusing in on the technological elements of it? Any thoughts on that question before we get to the closing question?
00:56:09 RASHIDA RICHARDSON: Sorry. Yeah, I’ll go. Yes, but I don’t think they have to be mutually exclusive, and I also don’t think we should delude ourselves into thinking that we can solve all bias and discrimination issues. Because there’s tons of research showing that implicit bias trainings don’t work. And it’s in part because it’s a lot of hard work to even recognize a lot of those powers and privileges that we have, and how to address them in meaningful ways.
So, I think part of the emphasis on the systems is because some of these can be fixed. Part of why I’m even interested in these issues is I think it’s going to force a conversation around the other issues we don’t want to deal with about bias in society. And then part of the reason it’s difficult is because it’s not an easy like oh you take the Harvard implicit bias test and then you can say I’m going to stop having a bias towards this group. It’s not that simple because a lot of bias, especially in the US context are structural and systemic, so it’s baked into the systems of government and our social systems, economic systems, it’s baked into the structure that we work within. It’s going to take a lot of work to help fix those problems, but I think the first step is just being more honest about the way that bias works within our society, and then trying to think about the solutions to these systems as addressing some of the structural and systems problems. Help bringing them to light to people who don’t understand they exist.
00:57:45 LUCY BERNHOLZ: And what could be more structural than baking this into the system, these decision-making tools that we don’t even see. So, here’s the last question. This has been a fabulous conversation. To all of the panelists and to the folks who’ve been submitting questions, we do want to keep this conversation going so please continue to send in the questions, the panelists will try to respond in text if they can. If there’s room for another conversation let us know, we’d be happy to have it.
But here’s the last question for our panelists which goes to this idea of how do we successfully interdisciplinize [phonetic] — that’s not a verb but I’ll make it one — and by that, I mean lived experience, academic experience, policymaking experience, experience experiencing a system, all that kind of expertise. Are there ideas, are there examples, things we can think of in the US and globally where we can do better? We may not do perfect, we [inaudible] perfect but we can do better. Each of you works in places that are trying to walk the talk, but what can you share with others who might be trying to put that into place?
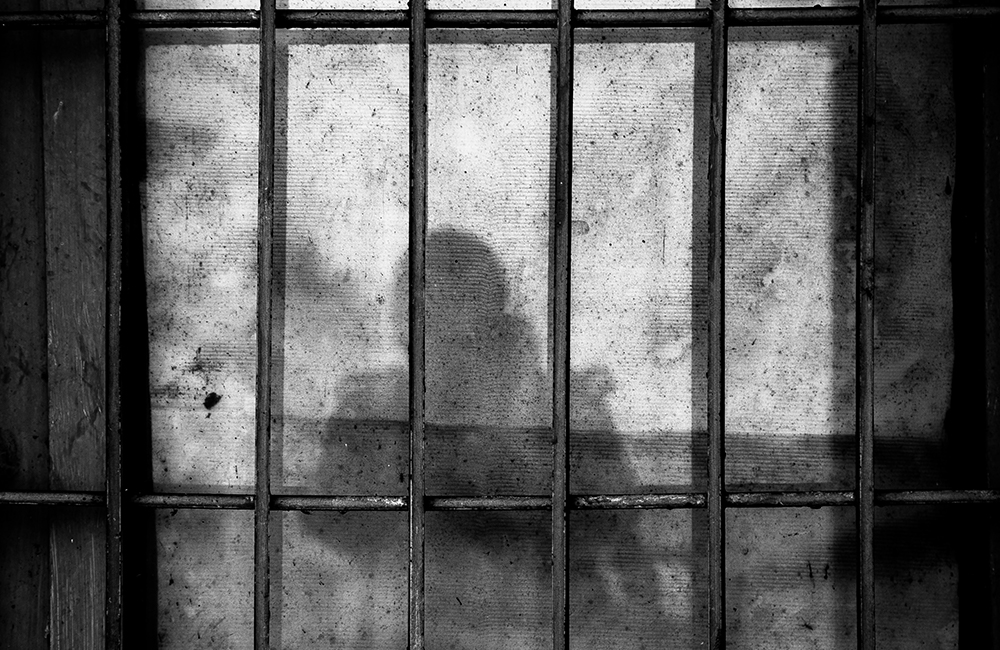
With the help of a Digital Impact grant, Media Mobilizing Project is charting the effects of predictive technologies on pretrial offenders. Ye Jinghan, Unsplash (CC BY 2.0)
00:58:54 VIRGINIA EUBANKS: I think those two questions are actually really related to each other. I think that, of course, we’re capable of and must do all of these things at once. We have a really hard cultural work to do. We have really hard political work to do, and we have really hard technological work to do. And we must and can do all those things at the same time, but part of doing that is really rethinking who experts are and what the decisions we’re making actually are rooted in. And I think they’re fundamentally rooted in values that we’re at a time in our history where we’re over-focused on the values of efficiency and optimization, and we’re missing some really important contextual values about dignity and self-determination and justice. And the folks who are experts in dignity and self-determination and justice need to be at the table when we have all of these conversations.
00:59:48 LUCY BERNHOLZ: Thank you.
00:59:51 RASHIDA RICHARDSON: Everything Virginia just said [laughs] plus I’d like to challenge an assumption that I think a lot of people make that this stuff is too difficult for people, like laypeople to understand, and it’s not. Because as we’ve been discussing a lot of these problems are a result of broader systemic and structural problems that tons of communities are very familiar with. And I think just because the issue in itself is technical we need to give society more credit and that people can understand, you just have to meet people where they’re at and explain it to them.
And that’s in part I guess what we’re trying to do as an organization is doing interdisciplinary work and meeting people where they’re at to make sure they’re informed enough to engage. Because some of the work is making sure people have access to information so they can meaningfully engage, and then also not assuming that you know everything.
Digital Civil Society Lab hosted Virginia Eubanks to explore the effects of automated decision making on government and the lives of citizens.
01:00:46 LUCY BERNHOLZ: Di, something to add?
01:00:47 DI LUONG: Yeah, I believe that our, the same rights that we have in real life should be carried over to our digital interactions, where it’s not enough to just have digital literacy, like downloading a VPN, using a Tor browser signal. It’s not the onus is on the individual but that we should have the right to know that our browsing data is not being sold to the highest bidder.
01:01:08 LUCY BERNHOLZ: Fantastic. Another important element of whose responsibilities are which because we’ve all got responsibilities here. I want to thank our panelists, this has been a fabulous conversation, thank the folks who were listening, and those who submitted questions. And thank the Mobilizing Media Project for helping to organize all of this.
If you missed any part of the discussion or want to share it with your colleagues, it will be available on the Digital Impact website and the Digital Impact podcast on iTunes, along with all of the resources mentioned.
Let’s talk about it. Comment below and tweet @dgtlimpact with your question or case study.
If you hosted a roundtable, what topic would you cover? Tell us here.
If you want to learn more about Virginia Eubanks or order a copy of her book Automating Inequality, visit Virginia-Eubanks.com. To see how AI Now Institute follows emerging technologies and their impact on diverse populations or to read the litigating algorithms report, visit AInowinstitute.org. And for a closer look at the Media Mobilizing Project and all the things they’re working on to address algorithmic bias in pretrial risk assessment, visit mediamobilizing.org. And please stop by Digital Impact for tips on how to advance the safe, ethical, and effective use of data in civil society.
I’m Lucy Bernholz at the Digital Civil Society Lab at Stanford PACS. Thanks for joining us. Goodbye for now.
Resources Mentioned in This Podcast
Shared by Virginia Eubanks
- Data justice project Our Data Bodies works with communities to investigate how information is collected, stored, and shared by government and corporations.
- The Seattle Surveillance Ordinance aims to provide greater transparency to the City Council and the public when the City acquires surveillance technology.
Shared by Rashida Richardson
- AI Now Institute’s Algorithmic Accountability Policy Toolkit provides legal and policy advocates with a basic understanding of government use of algorithms.
- AI Now Institute’s Litigating Algorithms Report explores current strategies for litigating government use of algorithmic decision-making.
- AI Now Institute’s Algorithmic Impact Assessments Report gives a practical framework for public agency accountability.
- AI Now Institute’s 2017 Symposium Report provides an update on the landscape of AI issues.
- Letter to NYC Automated Decision Systems Task Force: Recommendations for identifying automated decision systems in NYC government and developing solutions for mitigating harm
- Open Letter to Massachusetts Legislature: Informs the governing body’s consideration of risk assessment tools as part of ongoing criminal justice reform efforts in the Commonwealth
- Boston Magazine article about how the city’s new system reflects “a potentially troubling trend”
- DotEveryone article by Rashida Richardson on addressing bias: “Can Technology Help Undo the Wrongs of the Past?”
- Anatomy of an AI System: The Amazon Echo as an anatomical map of human labor, data and planetary resources
Shared by Di Luong
- Grantee Profile: Algorithmic Risk Assessment in Pretrial Detention
- Project Description: Media Mobilizing Project
- Newsweek op-ed by Media Mobilizing Project’s Hannah Sassaman on racist algorithms
- Bloomberg op-ed on how Philadelphia should think twice about its risk-assessment algorithm
- Boston Globe article on what happened when City Public Schools tried for equity with an algorithm
- Philadelphia community organizers oppose pretrial risk assessment plan
Shared by the Audience
- More than 100 orgs signed The Use of Pretrial “Risk Assessment” Instruments, a statement of rights concerns about the use of predictive tech in criminal justice.
- A People’s Guide to AI is a resource for understanding the impact of artificial intelligence on society.
- Mortgage lending in Philadelphia has brought attention to technology’s role in weakening the city’s race relations.